Data Analytics for Financial Services Providers
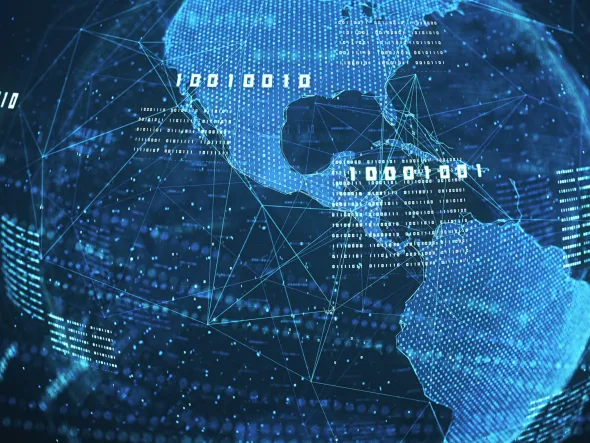
Data-analytics—or how to find the best uses for data-driven tools
How can banks and insurance companies have any certainty that new data-driven tools – like data analytics – will be successful? And how should they prioritize implementation? Our clients and our colleagues ask us these questions time and again. Our many client projects have taught us that all use cases have to be judged on their individual merits. Our exchanges with customers and other experts have allowed us to distil two essential steps for assessing use cases: first, determining the commercial value of each step being considered; and, second, identifying the factors that will raise the chance that chosen measures are implemented successfully.
One problem we encounter repeatedly is that financial-services companies often adopt ideas being used in other sectors without proper reflection or validation. These companies appear to be driven by the sentiment that other industries are more innovative, or by a more general, but diffuse passion for technological innovation. Big tech companies like Microsoft, Google and Microsoft are frequently held up as examples to emulate, but for the wrong reasons. Big tech companies’ data-driven approaches proved successful because they were responses to specific business problems. They knew one size does not fit all.
In light of this, banks and insurers should only pursue a data-driven solution if they are certain it will bring a material benefit – or what we call “realizable marginal gain”. On top of this, companies should not shy away from traditional cost-benefit analyses. Corporate cultures have recently taken a shine to ad hoc decision-making and ideas like failing forward in the name of being – or appearing – innovative. But cost-benefit analyses remain the most effective way to focus on and prioritize measures. They leave banks and insurance companies more time and money to allocate to the solutions most likely to succeed.
But not all use cases that show promise at this early stage deliver the hoped-for success. There is always the risk that projects will fail as they are being implemented. That is why we have identified five criteria to assess implementation risks: maturity is a measure of the company’s experience with introducing similar instruments; data for calibration a measure of the volume and quality of data underlying the planned data analysis; implementation teams a measure of the quality of interdisciplinary teams that also work with external advisers; selection of analysis method a measure of its suitability to solve the target problem; integration level a measure of the likelihood of results being accepted and used.
zeb’s two-step evaluation method has time and again helped our clients sift huge numbers of possible use cases to identify a handful of the most promising measures. For example, we once helped a financial-services company whittle down 300 potential use cases to eight very promising ones. Our method also gives employees involved with the assessments a structure for their work and allows management to clearly communicate its expectations. Both factors also help to markedly raise the quality of use cases eventually identified.