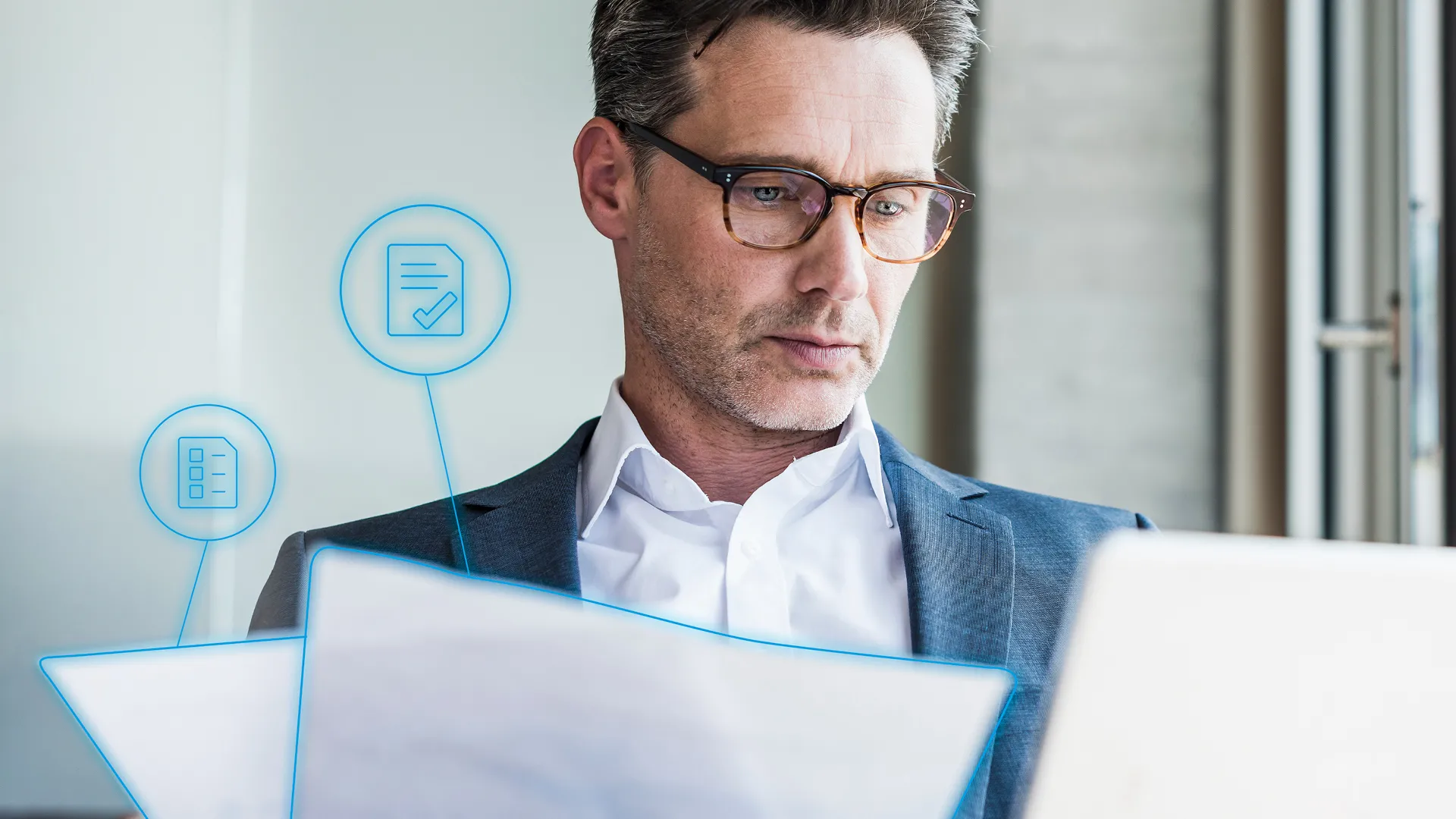
Designing efficient processes
AI brings old processes up to speed
AI brings old processes up to speed
Becoming efficient, staying fit for the future
In the past, banks and financial services providers were quite successful in optimizing their processes through step-by-step improvements or partly even through radical reorganization measures – this established their reputation as a pioneering industry in the field of IT. But nothing lasts forever: in order to regain momentum and reduce frictional losses in the value chains, banks must optimize their processes in a targeted manner using modern methods. If they don’t, they will fall even further behind their digital competitors.
Compared to other areas, especially the middle and back office still have significant potential to secure their performance and thus future viability. In this context, they should not only focus on technology, but also on breaking down barriers and preparing the ground for transformation.
To achieve competitive speed and efficiency, organizations need to intelligently automate their processes and make them part of the collective subconscious. Artificial intelligence and data analytics are perfectly suited to getting processes up to speed. An organization’s existing training plan can be intelligently expanded by automating more and more processes and intermediate steps that were previously performed manually.
AI and data analytics: advantages of optimized processes
Five application scenarios for AI methods
Using conventional methods from business process management, process quality can only be improved to a certain extent. This is due to organizational or systemic barriers in heterogeneous IT landscapes that have grown over time. These barriers can only be circumvented manually. Merging the different systems is either not possible at all or at least not in a cost-effective manner.
In such cases, efficiency can be increased with the help of robotic process automation (RPA). In addition, RPA reduces process cycle times as well as process errors. The targeted reduction of monotonous, manual tasks provides noticeable employee relief. This not only leads to a focus on value-adding activities, but also to increased employee satisfaction.
In order to optimize process steps using software-based methods the former must already digitized. OCR technology (Optical Character Recognition) is very helpful in that regard, as it can be used to extract texts from images and documents. Repetitive, manual and paper-heavy business processes involving data extraction from documents are ideal for this. A typical case is the recognition and extraction of information from manually filled forms. This allows analog formats to be made available in digital form. Then, with the help of further AI methods, the efficiency of subsequent process steps can be increased.
For paper-heavy business processes, documents not only have to be transferred from analog to digital formats, but the information provided also has to be further processed based on context. To achieve this, documents must also be categorized. Categorization is another machine vision technology that allows images or data to be classified into categories based on certain features or characteristics. Besides the classic use case in document submission for checking the completeness of documents, this technology can also be used in the area of fraud detection. For example, categorizing transaction data makes it possible to analyze patterns and detect fraudulent activity.
Machine processing and generation of natural text is a classic AI application. There are many suitable use cases at the customer interface, as well as in the mid- and back-office. While the focus at the customer interface is on direct dialog, the focus in the mid- and back-office is on processing large amounts of text to extract information. These texts may include many different types of documents such as, for example, press releases, financial and business documents, e-mails, contracts or presentations.
A special type of classification is known as sentiment analysis. Such an analysis can give financial services providers valuable insights as to how their product, their company or a third party is perceived. Another application closely related to this is the generation of natural language text from structured data. The challenge in this application is to make the text not only informative, but also emotional. Currently, automated text generation is used where the focus is on quantity and efficiency rather than on particularly high quality.
Each cycle of a process generates data on the process itself. These traces allow others to track the steps employees or customers have taken in the application system. Based on this real-world generated data, process mining allows the actual process cycles to be compared to the intended plan – which often differ quite a lot. This reveals where the bottlenecks in the process are. Ideally, in order to pursue a holistic approach, the process data should be merged and evaluated across system boundaries. This requires a comprehensive overview of all processes within the company. Furthermore, the processes should be well-structured and well-modeled, and each individual step should be available to be read out by the IT system. The most decisive success factors in that respect are the quality, variance and volume of the available data. Here, the old saying applies: a little is good, a lot is better.
AI is not a question; it is the answer to profound changes in the market for financial services providers. Do you want to make your processes more efficient? We look forward to helping you tackle your challenges!